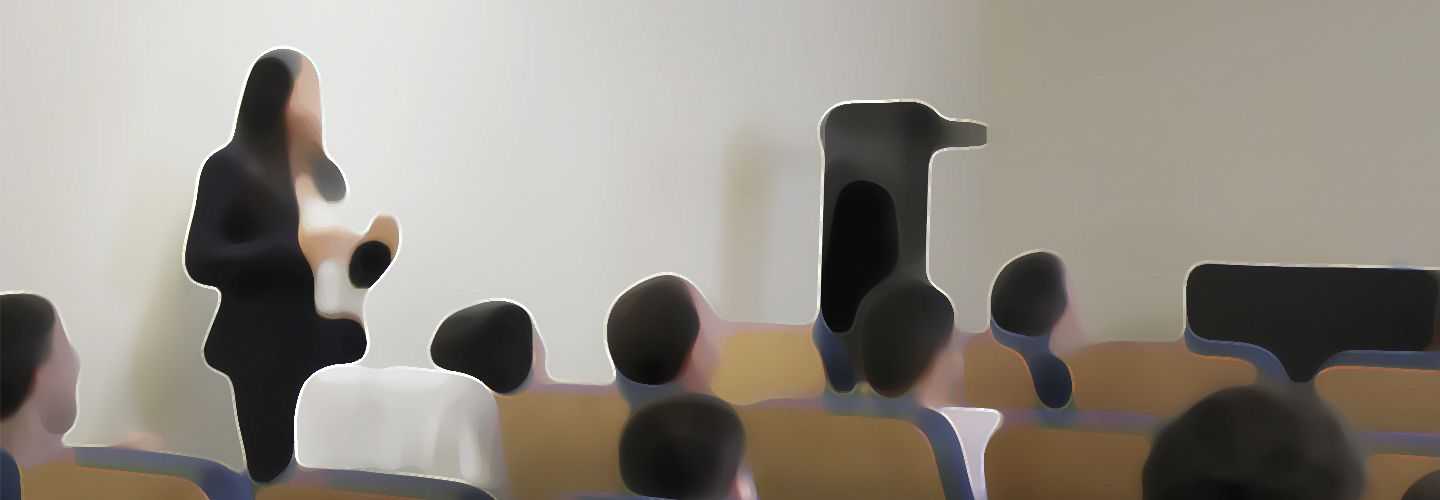
Research Insider: '3D Cardiac Image Analysis Using Machine Learning and Classic Techniques: Challenges and Possibilities'
Many aspects related to the pathogenesis and progression of cardiac arrhythmia are still not fully understood. Besides, current risk stratification techniques and clinical treatments have shown sub-optimal success rates showing the need for developing novel research approaches and computational methodologies. Non-invasive imaging techniques (e.g., MRI, CT) have emerged as powerful tools to identify structural changes of the heart, including detailed cardiac tissue characterization.
Machine learning (ML) techniques have shown to be powerful tools to perform automatic segmentation, classification, predictive analysis, etc. in many fields. Unfortunately, the application of ML to cardiac imaging data poses several challenges. In particular, the non-standard nature of the data being multimodal, multiscale, longitudinal, highly variable (the heart is beating and the patient is breathing during the imaging study, every heart is different (shape, function, electrical properties...)) makes it particularly challenging to process a high number of samples fully automatically. In this talk, I am going to present some of my work related to the processing, analysis, and standardization of cardiac imaging data, and I am going to show several case examples of how our methods have been included in clinical research practice.
Bio
Marta Nuñez-Garcia has recently joined the CiTIUS as a postdoctoral researcher. She holds a Master's in Computer Vision and Artificial Intelligence from the Universitat Autònoma de Barcelona (Spain), she received her PhD from the Universitat Pompeu Fabra (Barcelona, Spain), and she worked as a postdoctoral researcher at the IHU-Liryc (Université de Bordeaux and Inria) in France for 4 years. She investigates the use of machine learning techniques and classical image processing approaches applied to multimodal cardiac images such as MRI, LGE-MRI, CT, LIE-CT, etc. Her main research interest focuses on developing standardized multimodal and multiscale cardiac models for the statistical analysis of cardiac data using big datasets and machine learning-based methods. The aim is to better understand the mechanisms that trigger arrhythmia and other cardiac diseases, and to improve current risk stratification techniques, treatment planning and patient care.
Evento mixto
/events/research-insider-3d-cardiac-image-analysis-using-machine-learning-and-classic-techniques-challenges-and-possibilities
events_es