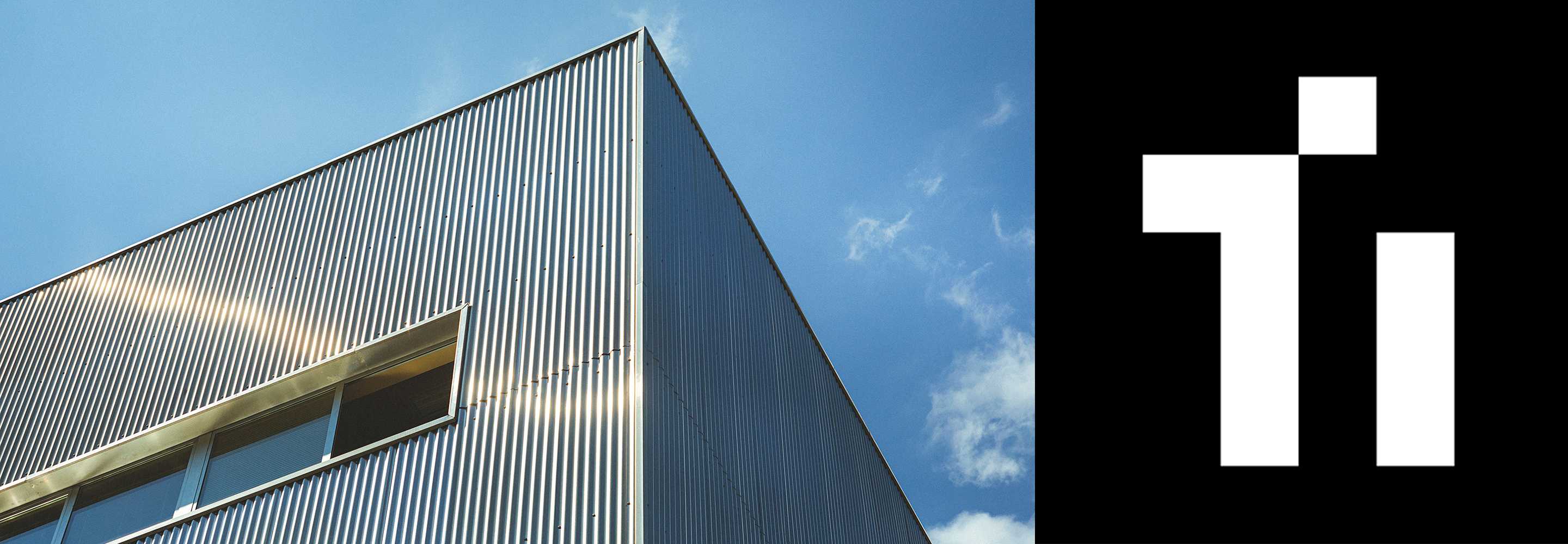
Doctoral meeting: 'Argumentative counterfactual explanation generation for enhancing human-machine interaction'
Recent years have witnessed a rapid growth of interest in automatic explanation generation for artificial intelligence applications. Thus, the obscure nature of various machine learning algorithms may decrease users’ trust in their predictions unless they are thoroughly explained. Moreover, legal regulations impose rigorous requirements on intelligent systems making use of algorithms with a poor self-explanatory capacity.
It appears particularly useful yet challenging to explain a given algorithm’s output in terms of reasonable but non-predicted alternatives. Further, such so-called counterfactual explanations suggest minimal changes in feature values enabling the end-user to obtain a desired outcome instead of the predicted one. While providing the user with means for constructing a big picture of the reasoning behind the given algorithm, counterfactual explanations should be properly communicated to and questioned by the enduser if found necessary.
This doctoral meeting aims to (1) give a brief overview of state-of-the-art methods for counterfactual explanation generation, (2) describe the proposed method of counterfactual explanation generation for decision trees and fuzzy rule-based classification systems, (3) propose an argumentation-based dialogue protocol for communicating automatically generated factual and counterfactual explanations as well as (4) outline main challenges to be addressed as part of future work.
Supervisors:Jose M. Alonso, Alejandro Catalá and Martín Pereira-Fariña
Virtual event
/events/doctoral-meeting-argumentative-counterfactual-explanation-generation-for-enhancing-human-machine-interaction
events_en