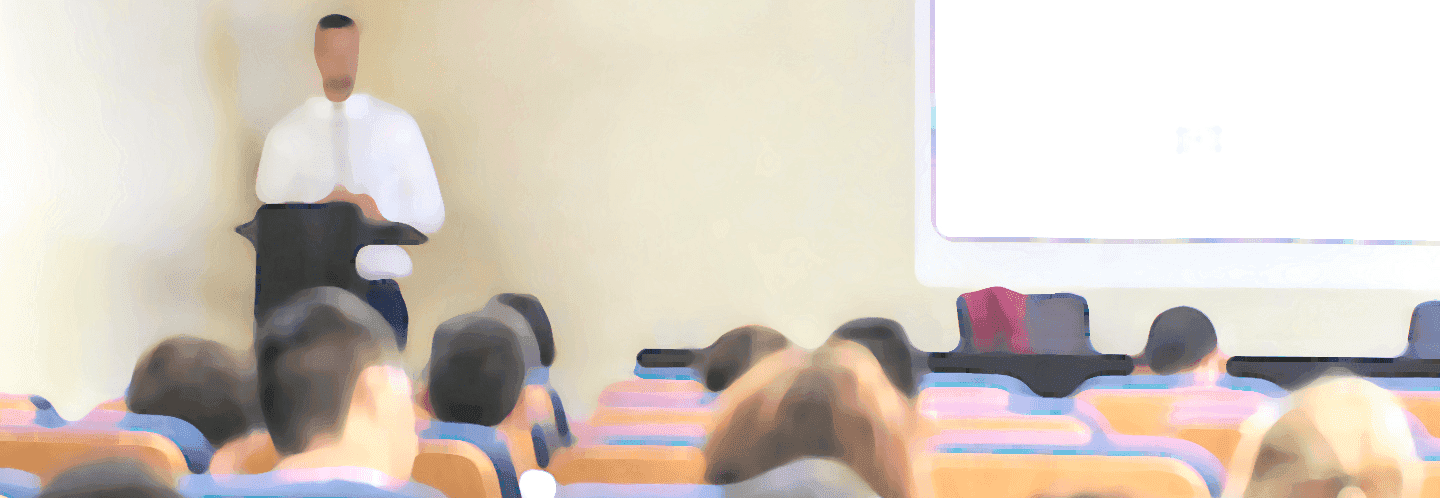
Doctoral meeting: 'Bayesian nonparametric dynamic clustering of time sequences'
Time series analysis has assumed an increasingly central role in machine learning. One of the main challenges in this context is the dynamic nature of time series data, which often exhibits non-stationary patterns over time. Traditional clustering techniques, primarily designed for static data, fall short when confronted with such dynamic temporal behaviour.
Our proposal is a Bayesian nonparametric model based on Gaussian Processes and Linear Dynamical Systems, which can identify evolving morphologies, reducing the number of generated clusters. Inference tools were developed based on a variational inference approach. A testing phase was conducted on the MIT-BIH Arrhythmia Database, achieving a reduction in the number of clusters compared to the state-of-the-art method. One of the biggest advantages of our model is the interpretability of the results, not only easing the understanding of the data dynamics but also leading to new insights related to the prediction of the breathing signal using the ECG.
On-site event
/events/doctoral-meeting-bayesian-nonparametric-dynamic-clustering-of-time-sequences
events_en