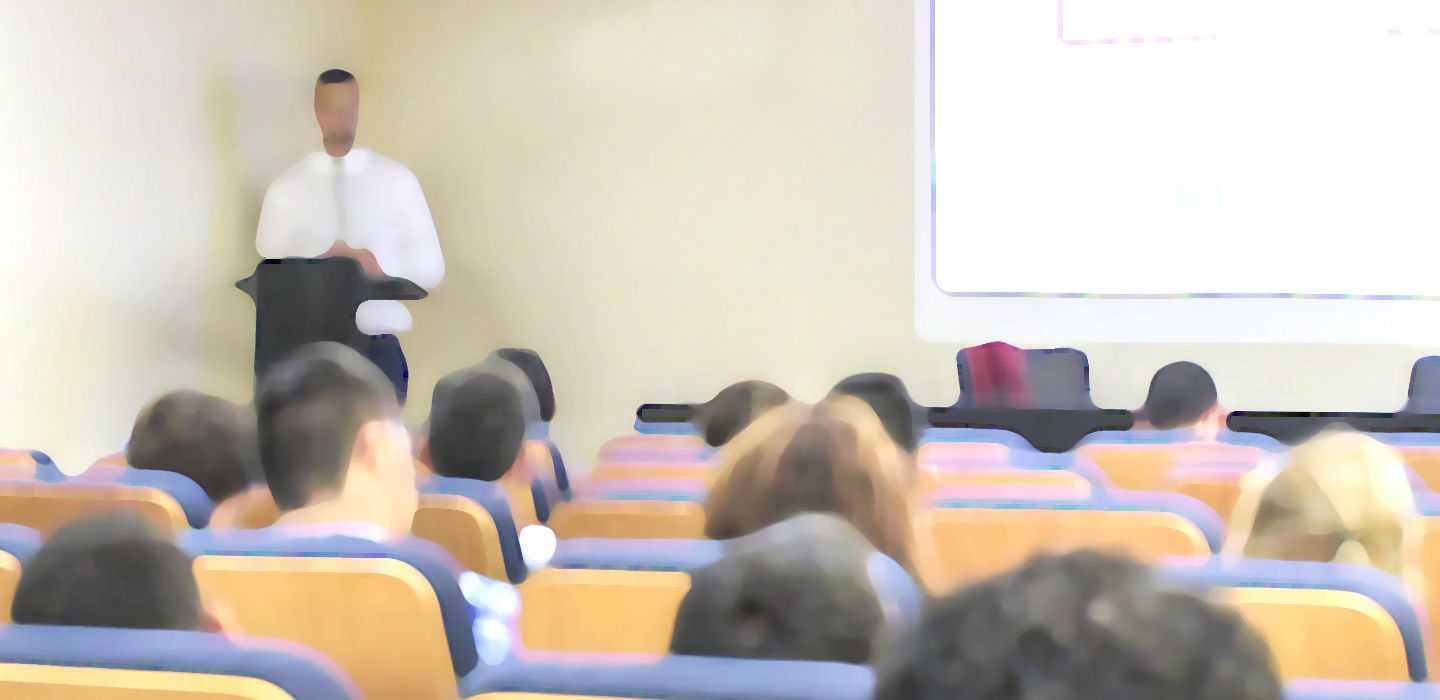
Doctoral Meeting: 'Dealing with hallucinations and omissions in neural Natural Language Generation'
The current trend to address the Natural Language Generation (NLG) task is to use neural models trained with millions of textual datasets and corpora, e.g., GPT-4, T5, BERT, etc. These models are optimized to generate natural and fluent texts, similar to the ones a human would write. However, in many cases they generate divergences with respect to the input data, either in the form of omissions or hallucinations. Our research focuses on detecting and evaluating such phenomena for the data-to-text task, specifically in the automatic generation of weather forecasting texts from structured data. We trained different neural NLG models to accomplish the weather forecasting generation task and analyzed the readability and content correctness of the generated texts using different tools.
Supervisor: José María Alonso Moral
Mixed event
/events/doctoral-meeting-dealing-with-hallucinations-and-omissions-in-neural-natural-language-generation
events_en