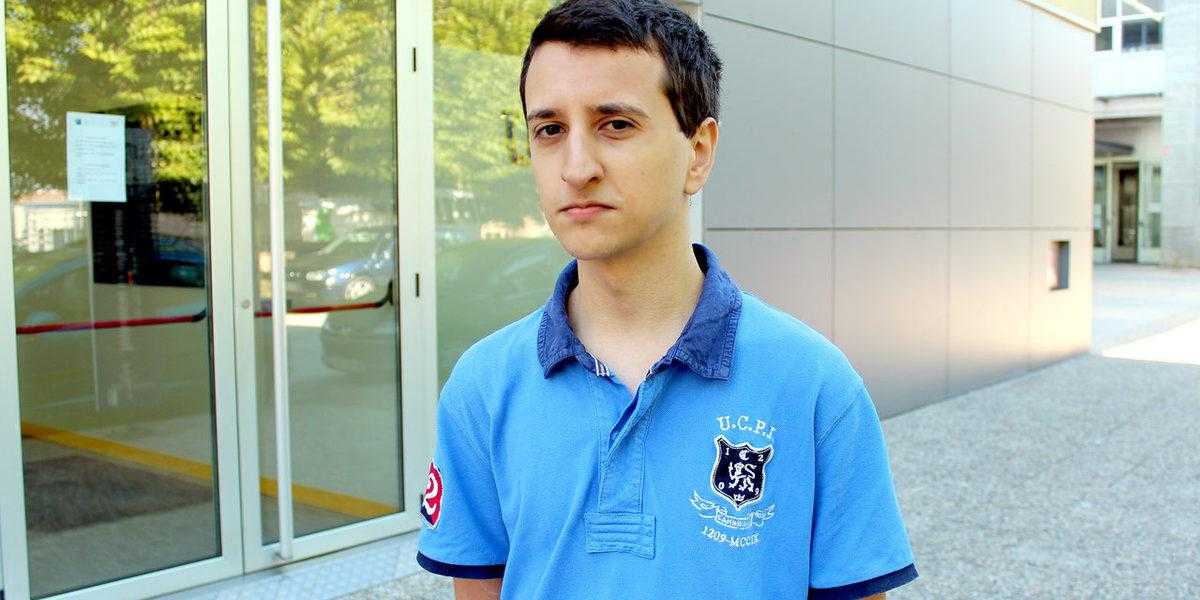
Doctoral meeting: 'Deep learning for predictive process monitoring based on change characterization and detection'
Process mining is an area of business intelligence focused on the analysis and improvement of business processes, using as inputs process logs that store the traces of processes executions. The objective of process mining is understanding what is really happening instead of what is believed to be happening. For that, in process mining we distinguish three types of basic techniques: process discovering, where a process model is obtained based on the behavior observed in process logs; process enhancement, where the process model is enhanced with additional information that enriches its description; and process conformance checking, where we evaluate whether the behavior observed in the process logs can be reproduced by process models and to measure the differences between them.
Thus, process conformance checking pretends to explain what has happened, helping to understand how to improve business processes. However, in domains where resource management has an important impact in the business process, the need for predicting business process behavior is imperative, i.e, in what measure some relevant parameters are adjusted to expectations. This is the main objective of what is known as predictive process monitoring, the research line in which the current project is focused.
The prediction of these parameters of the business process is very useful for organizations that need to plan resources or activities in the medium/long term. For example, predicting that in the next two weeks a certain path of the process models is going to be executed 30% of the time will ease the management of the resources of the organization which participates in the activities of the path. However, most approaches for predictive monitoring are focused on predicting only what happens in the context of the execution of a trace, even though we need to predict multiple traces in a certain time interval to allow making the planning previously described. Furthermore, the codification scheme of the traces used by these approaches is very simple, which makes the prediction results unstable. On the other hand, we will need to detect and characterize the possible changes in the business process so as to improve even more the reliability of predictions.
This project pursues a step forward in the predictive monitoring field combining trace prediction techniques to medium/long range with techniques for detection, characterization, and prediction of changes in business processes. Furthermore, the algorithms developed in the project will be based in deep learning with an encoding scheme that will improve trace characterization, allowing managing both its heterogeneity and the massive amount of traces generated in the domains of interest.
Supervisors: Manuel Lama Penín and Juan Carlos Vidal
Virtual event
/events/doctoral-meeting-deep-learning-for-predictive-process-monitoring-based-on-change-characterization-and-detection
events_en