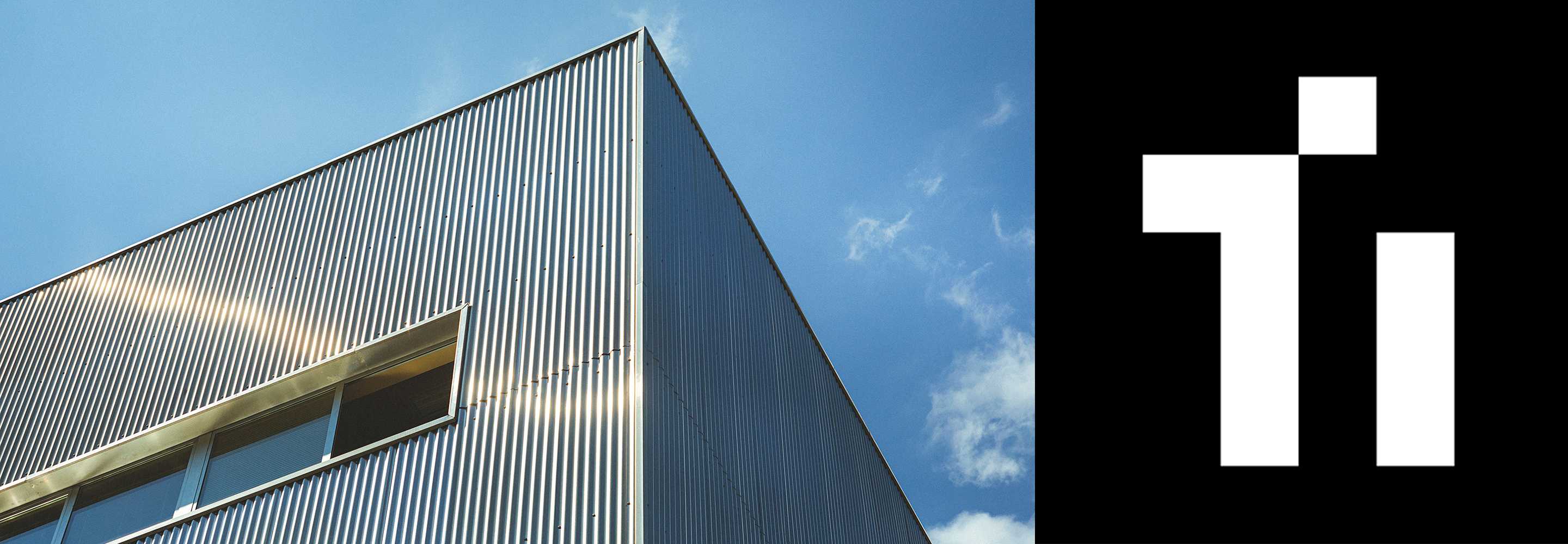
Doctoral meeting: 'Development of a 'glocal' and continuous machine learning strategy applicable to devices'
Today we live in a context in which devices are increasingly interconnected and sensorized and are almost ubiquitous. Deep learning has become in recent years a popular way to extract knowledge from the huge amount of data that these devices can collect.
Nevertheless, state-of-the-art learning methods have several drawbacks when facing real distributed problems, in which the available information is usually partial, biased, and changing over time. Moreover, if there is something that characterizes this society of devices is its high heterogeneity and dynamism, both in terms of users and the hardware itself.
Therefore, against the tendency to centralize learning, we aim to develop a new paradigm of learning in society, where devices get certain prominence back, having to learn in real time, locally, continuously, autonomously and from users, but also improving models globally, in the cloud, combining what is learned locally, in the devices.
In this presentation we will delve into our first architecture for this paradigm, which we call "glocal" learning.
Moderator: Dora Blanco
Supervisors: Roberto Iglesias and Senén Barro
Mixed event
/events/doctoral-meeting-development-of-a-glocal-and-continuous-machine-learning-strategy-applicable-to-devices
events_en