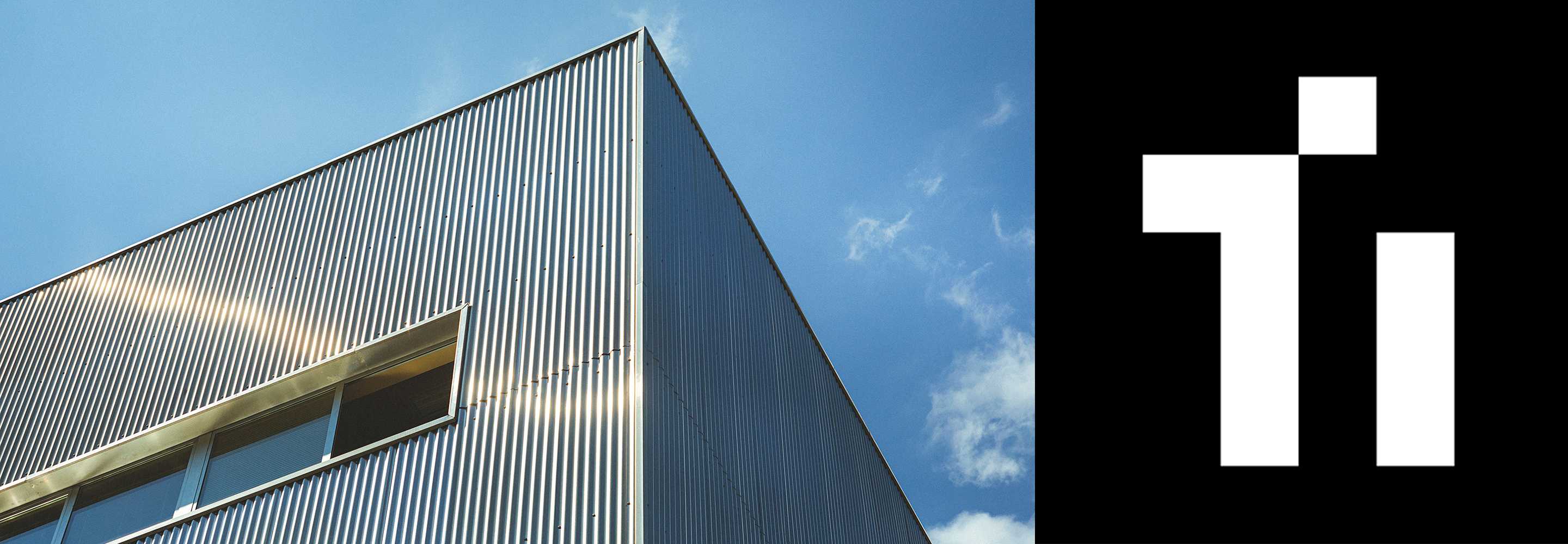
Doctoral meeting: 'Five-year prediction of glucose changes with missing data in a Reproducing Kernel Hilbert Space'
Diabetes is one of the most critical public health problems worldwide. It is currently estimated that around 50% of people with type II diabetes are undiagnosed. In this context, intelligent technologies can be an essential weapon to improve clinical decisions, specifically for early detection of the disease and identifying phenotypes of patients that need further longitudinal follow-ups over time.
With these applications in mind, in this talk, we will introduce one of my Ph.D. works in which new kernel methods are proposed when the response variable is missing in some individuals. In particular, new statistical independence tests, variable selectors, and procedures to quantify uncertainty are proposed. The different techniques are illustrated in the problem to model long-term glucose changes.
Supervisors: Paulo Félix and Balbina Casas
Virtual event
/events/doctoral-meeting-five-year-prediction-of-glucose-changes-with-missing-data-in-a-reproducing-kernel-hilbert-space
events_en