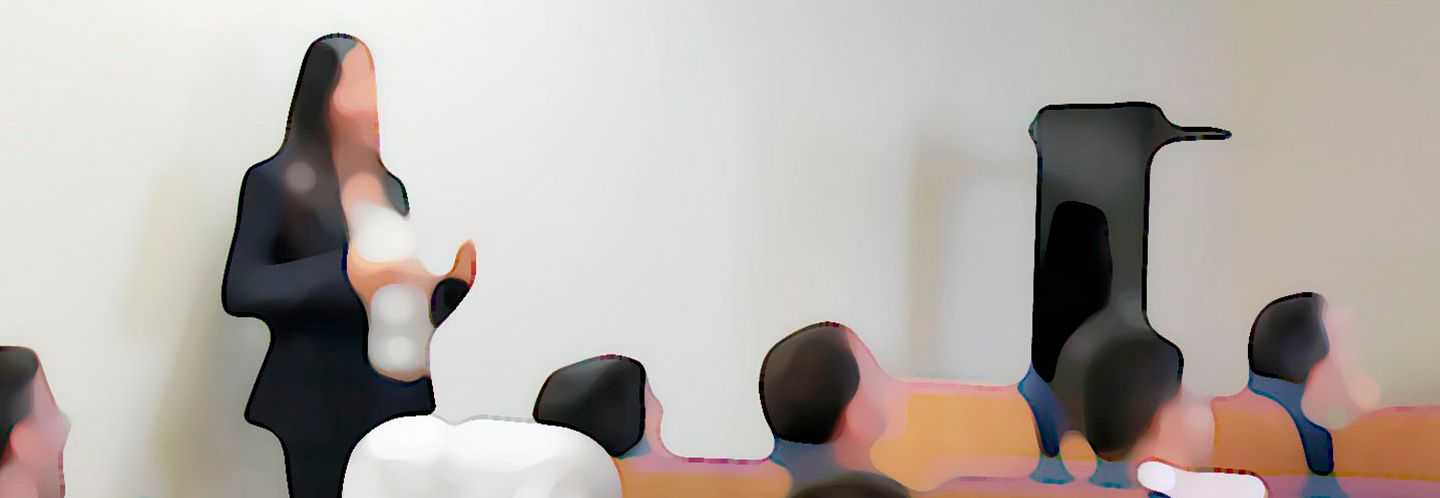
Doctoral Meeting: 'In-Memory Computing CMOS Circuits for Computer Vision Deep Learning Models'
In the domain of edge computing applications, hardware accelerators play a pivotal role in enabling real-time processing, with a particular focus on convolutional neural networks. Addressing the critical challenge of achieving low power consumption while maintaining performance accuracy is essential. This study comprehensively analyzes potential architectures for the core cell of the multiply-and-accumulate function. Each structure's essential advantages and limitations are analyzed through electrical simulations, presenting insights into their performance within a 180 nm process node at 1.8 V and 3.3 V.
Supervisors: Fernando Pardo Seco and Daniel García Lesta
On-site event
/events/doctoral-meeting-in-memory-computing-cmos-circuits-for-computer-vision-deep-learning-models
events_en