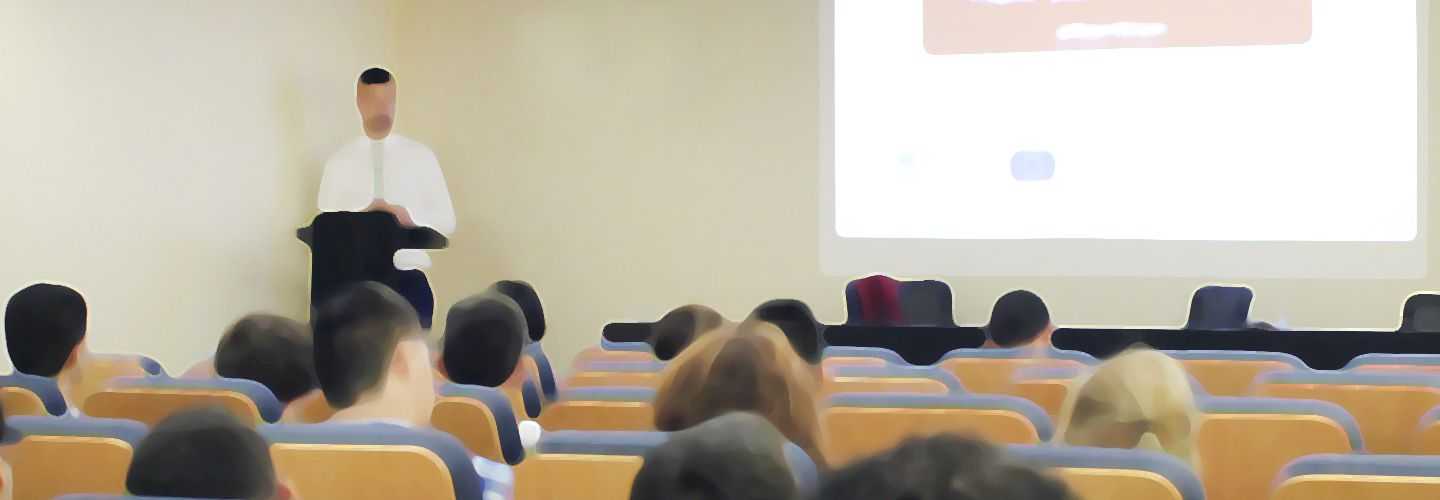
Doctoral Meeting: 'Latest research in Explainable Artificial Intelligence (XAI)'
(Part 1) 'Focus and Bias: Rating XAI Methods and Finding Biases'' (Anna Arias Duart)
The evaluation of the explainability techniques is a challenge. To trust the explanations, we must first check that they reliably approximate the model behaviour. This becomes a complex task due to the lack of a ground truth specifying what defines a correct explanation. Even if different feature attribution methods have been proposed in the literature, there is no standardization of the metrics to assess and select these methods. In our work, we offer an evaluation score for feature attribution methods designed to quantify their coherency to the task. We call it the Focus. To calculate this score, we generate mosaics composed of instances from different classes, and we apply, on top of them, the explainability method under assessment. The Focus metric will measure the proportion of attribution lying on the correct squares with respect to the total mosaic attribution.
On the other hand, explainability can also be used for bias detection. This process typically consists of a domain expert visualizing all the explanations in order to find unwanted biases. However, the vast amount of samples the domain experts must review makes this task more challenging as the dataset grows. In an ideal case, the system should provide domain experts only with a small number of selected samples containing potential biases. Since the Focus errors may correspond to visual biases in the model, the Focus score seems to be a promising tool for the selection of those samples containing potential unwanted biases.
About Anna
Anna Arias Duart obtained a Bachelor's degree in Telecommunications Technology and Services Engineering in 2015 from the Universitat Politècnica de València (UPV). In 2018 she completed the Double Diploma awarded by the UPV and Télécom ParisTech (Paris). She is currently a student of the Doctoral Program in Artificial Intelligence at the Universitat Politècnica de Catalunya within the Industrial Doctorate Program in collaboration with SEAT, S.A. Her research is focused on Explainable Artificial Intelligence (XAI) and more specifically on the explainability of neural networks.
(Part 2) 'XAI for time series data' (Natalia Jakubiak)
The ability to apply AI approaches to solve various problems in many industrial areas has been mainly achieved by increasing model complexity and the use of various black-box models that lack transparency. In particular, deep neural networks are great at dealing with problems that are too difficult for classic machine learning methods, but it is often a big challenge to answer the question why the neural network made such a decision and not another. The answer to this question is extremely important to ensure that ML models are reliable and can be held liable for the decision-making process. Over a relatively short period of time a plethora of methods to tackle this problem have been proposed, but mainly in the area of computer vision and natural language processing. Few publications have been published so far in the context of explainability in time series. This talk aims to provide an overview of the research in XAI for time series data as well as to present a solution to achieve and evaluate local explainability for a model in time series forecasting problem. The solution involved framing a time series forecasting task as a Remaining Useful Life (RUL) prognosis for turbofan engines.
About Natalia
Natalia is a member of the Knowledge Engineering and Machine Learning Group and Master’s degree student major in Artificial Intelligence at the Polytechnic University of Catalonia. She has been a research assistant since 2019. She has been researching in projects related to the application of Artificial Intelligence in areas such as Cybersecurity and Manufacturing.
Mixed event
/events/doctoral-meeting-latest-research-in-explainable-artificial-intelligence-xai
events_en