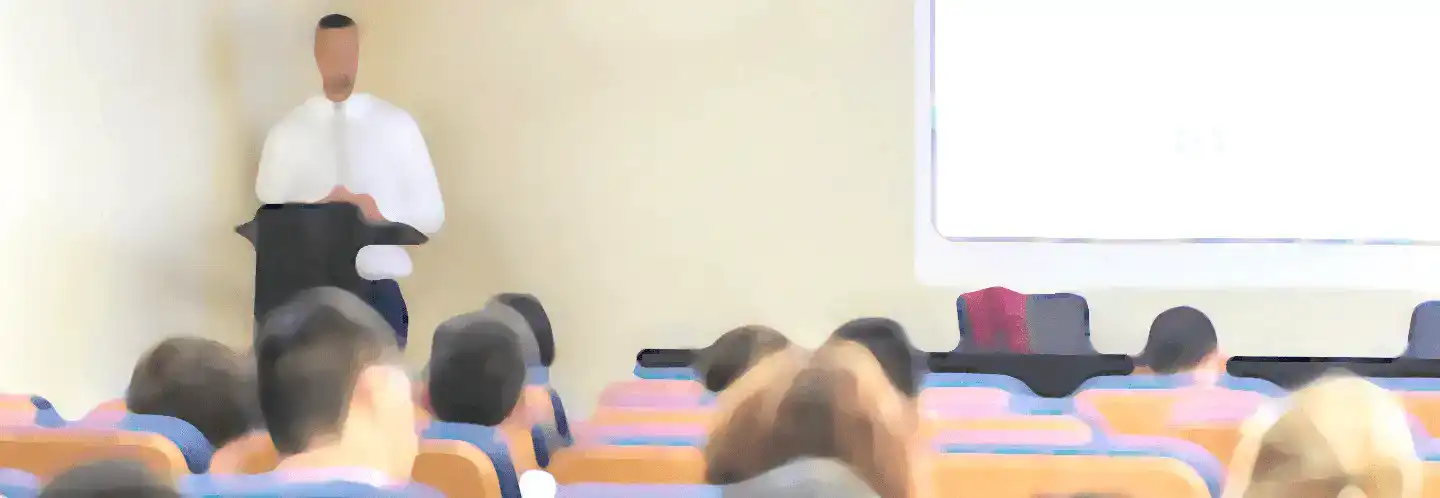
Doctoral Meeting: 'Machine Learning Techniques for Prenatal Detection of Small and Vulnerable Newborns'
Babies born small and vulnerable (SVN) face higher risks of mortality and health complications, including future heart disease. Current detection models typically focus on isolated risk factors rather than evaluating the fetus as a whole, which can result in missed indicators of growth restriction. Fetal growth is typically estimated using ultrasound-based measurements such as the length of the femur and the abdominal and head circumferences. Therefore, the first step towards improving diagnosis involves the accurate segmentation of these anatomical structures in fetal ultrasound images. For this purpose, we employ established architectures, such as UNet and nnUNet, for their demonstrated efficacy in achieving state-of-the-art results in the field. Additionally, we explore modifications to these base architectures to leverage structural similarities between the head and abdomen, further enhancing segmentation performance. As a future work, the computed fetal growth markers will be combined with multimodal data -including demographic and medical records from the mother- and population data- aiming at establishing a rigorous, safe, and unbiased framework for fetal growth assessment, that may lead to more reliable prenatal SVN diagnosis.
- Supervisors: Marta Núñez García and Nicolás Vila Blanco
- Moderator of this DM: Pablo Gamallo
On-site event
/events/doctoral-meeting-machine-learning-techniques-for-prenatal-detection-of-small-and-vulnerable-newborns
events_en