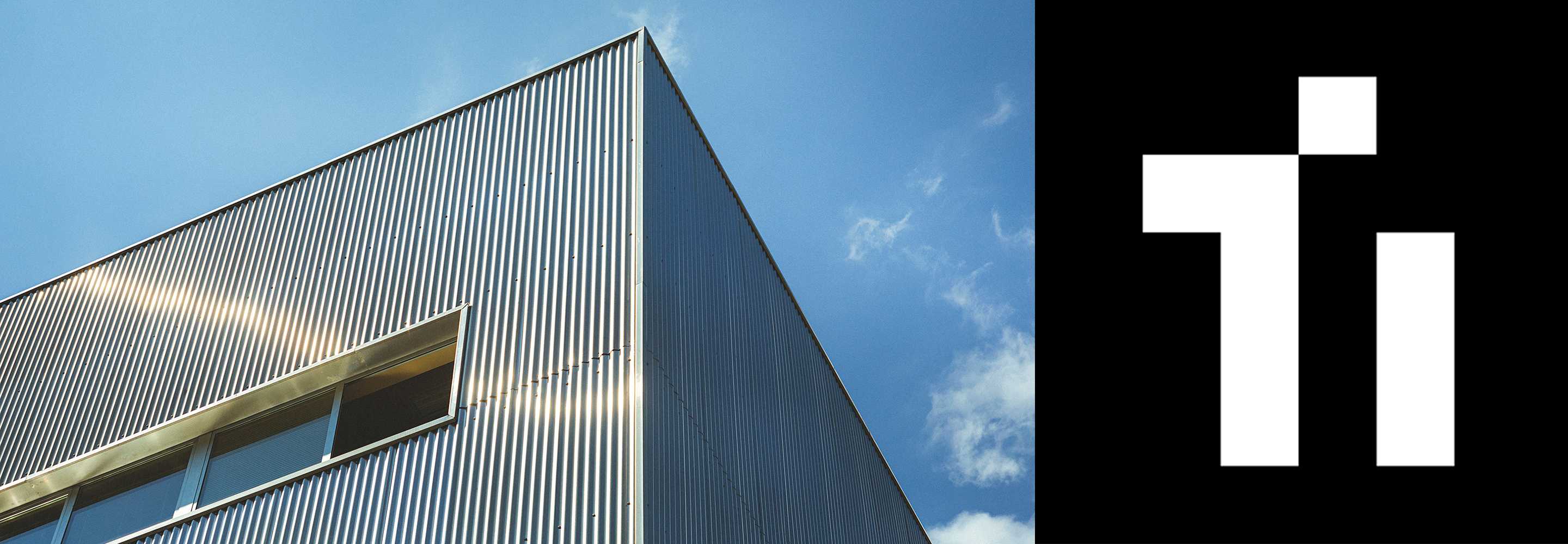
Doctoral meeting: `Techniques for the extraction of spatial and spectral information in the supervised classification of hyperspectral imagery for land-cover applications´
Hyperspectral and multispectral image capture technology advances have improved the data acquisition capabilities of the sensors used both in satellites and, more recently, in drones. Hyperspectral and multispectral images have been successfully used to perform a variety of tasks such as biodiversity studies, vegetation inventory management, etc. in a semi-automated manner or to increase the efficiency of productive processes in fields such as precision agriculture.
Land-cover classification is a supervised learning problem where we learn multiple classes from a training dataset comprised of a subset of pixels from an image. Hyperspectral images are computationally expensive to process due to the high volume of data they contain and the complex processing tasks involved, which requires specific mitigation strategies when attempting to perform land-cover classification.
In this thesis, we propose different approaches to reduce the computational time and increase the precision metrics for land-cover classification tasks involving hyperspectral and multispectral images. In particular, our efforts have focused on the efficient extraction of spatial and spectral information for traditional classifiers, and data augmentation techniques used in combination with neural network classification schemes.
Supervisors: Dora Blanco and Francisco Argüello
Virtual event
/events/doctoral-meeting-techniques-for-the-extraction-of-spatial-and-spectral-information-in-the-supervised-classification-of-hyperspectral-imagery-for-land-cover-applications
events_en