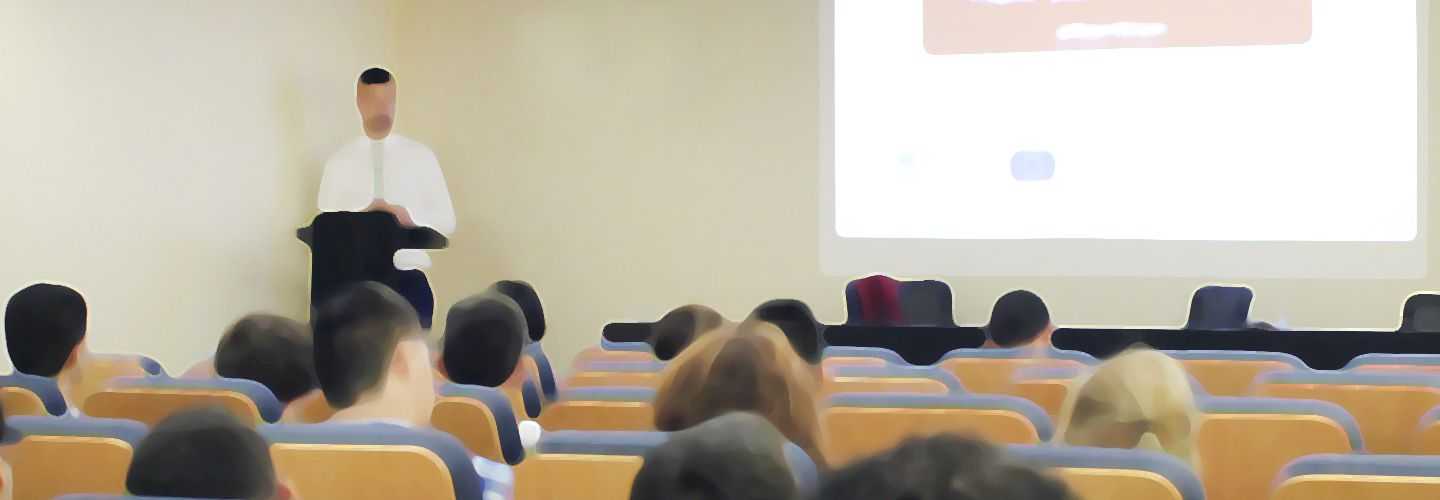
PhD Defense: 'Continual federated machine learning under concept drift'
Federated learning (FL) is a machine learning paradigm that allows training models in a distributed way, among multiple devices, without compromising user privacy. In this Ph.D. thesis, we propose new FL strategies that, while maintaining all the advantages that this technology already provides, also allow us to handle continual scenarios with non-stationary data and concept drift. We formulate two complementary strategies: CDA-FedAvg, which enables the training of a global deep neural network, and ECFL, which poses the model as an ensemble of local learners. Both approaches, CDA-FedAvg and ECFL, allow the temporal evolution of the models, detecting and adapting to concept drift. They also provide a reduction in storage, computation, and communications. We evaluated our solutions in different use cases, including human activity recognition in smartphones and navigational assistance to robotic wheelchair users. The results highlight the relevance and applicability of continual FL to real-world problems and, in particular, the advantages of our contributions.
Mixed event
/events/phd-defense-continual-federated-machine-learning-under-concept-drift
events_en