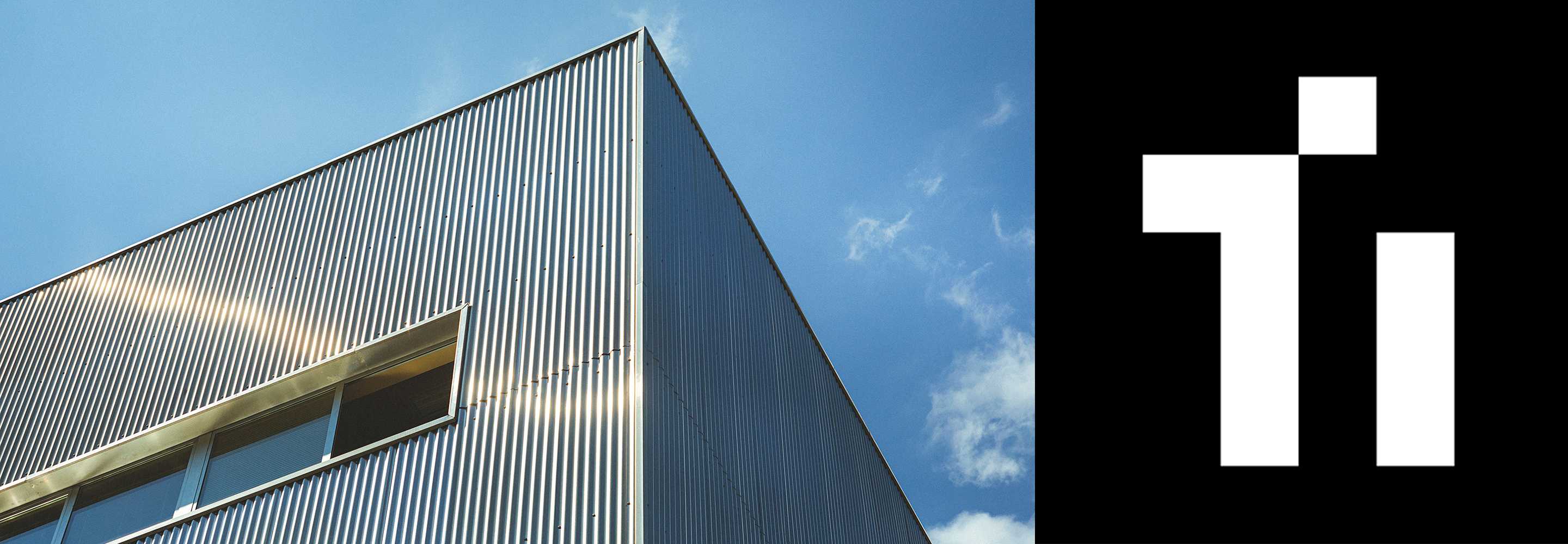
PhD Defense: ‘Ensembles of choice-based models for recommender systems’
In this thesis, we focused on three main paradigms: Recommender Systems, Decision Making, and Ensembles. For this purpose, first, the thesis analyzes the potential of choice-based models. The motivation behind this was based on the idea of applying sound decision-making paradigms, such as choice and utility theory, in the field of Recommender Systems. Second, this research analyzes the cognitive process underlying choice behavior. On the one hand, neural and gaze activity were recorded experimentally from different subjects performing a choice task in a Web Interface. On the other hand, cognitive were fitted using rational, emotional, and attentional features. Finally, the work explores the hybridization of choice-based models with ensembles. The goal is to take the best of the two worlds: transparency and performance. Two main methods were analyzed to build optimal choice-based ensembles: uninformed and informed. For the first one, two strategies were evaluated: 1-Learner and N-Learners ensembles. For the second one, we relied on three types of prior information:
- High diversity
- Low error prediction (MSE)
- Low crowd error. A Greedy approach was used to select the single models and to construct the ensembles.
Supervisor: Eduardo Sánchez Vila
Mixed event
/events/phd-defense-ensembles-of-choice-based-models-for-recommender-systems
events_en