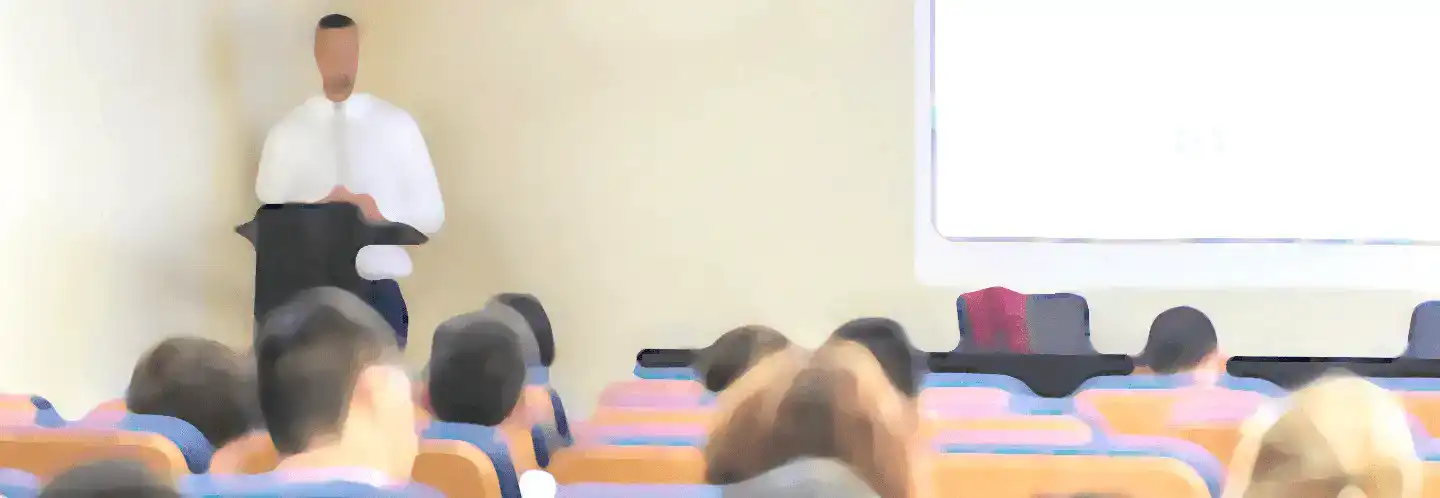
PhD Defense: 'Opening the AI Black Box: Advances in eXplainable Artificial Intelligence (XAI)'
Artificial Intelligence (AI) is transforming various industries, but many advanced models operate as "black boxes", making decisions that are difficult to interpret. This poses challenges in terms of transparency, trust, and accountability, underlining the need for more explainable AI.
This thesis addresses this problem through the lens of eXplainable Artificial Intelligence (XAI). Our objective is to explore how transparent models can act both as self-explanatory systems and as tools to explain the behavior of more complex systems. We present CNAM, a model that balances interpretability and performance, and new metrics based on Shapley values, such as Shap Length and Shap Gap, to measure model complexity and explanation fidelity. We develop the TextFocus frameworks to evaluate the fidelity of post-hoc explanation methods. We demonstrate the practical value of XAI through use cases at INDITEX, applying both white-box models and black-box explanations to uncover complex patterns and improve efficiency and transparency in key processes.
This work represents a step towards democratizing AI, making complex models more accessible, understandable, and trustworthy, with the potential to transform how we interact with AI and foster responsible innovation.
Supervisors: José María Alonso Moral and Albert Gatt
On-site event
/events/phd-defense-opening-the-ai-black-box-advances-in-explainable-artificial-intelligence-xai
events_en