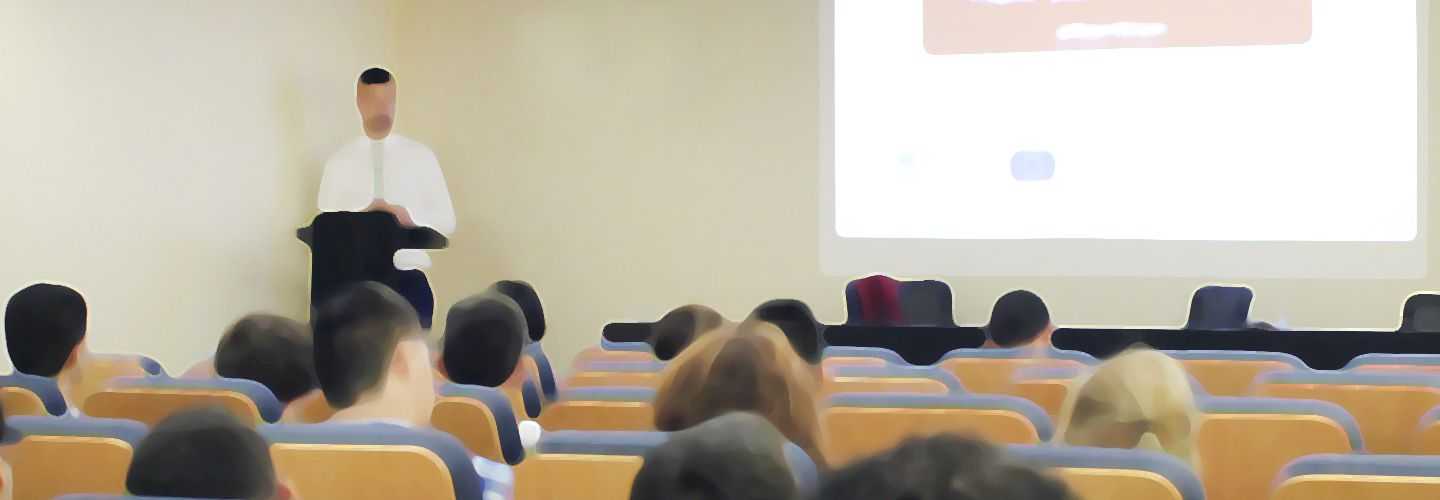
PhD Defense: 'Spatio-temporal convolutional neural networks for video object detection'
The precision of object detectors in images has greatly improved with the use of Deep Learning techniques, especially with the adoption of Convolutional Neural Networks. However, object detection in videos presents new challenges such as motion blur, out-of-focus or object occlusions that make object detection more difficult. This thesis proposes new methods to exploit spatio-temporal information, establishing relations among detections from different frames and performing feature aggregation throughout time. This improves the detection precision in frames in which a single image object detector would not be able to provide the correct object category. This thesis also explores the utility of spatio-temporal information to reduce the number of training examples, keeping a competitive detection precision.
Mixed event
/events/phd-defense-spatio-temporal-convolutional-neural-networks-for-video-object-detection
events_en