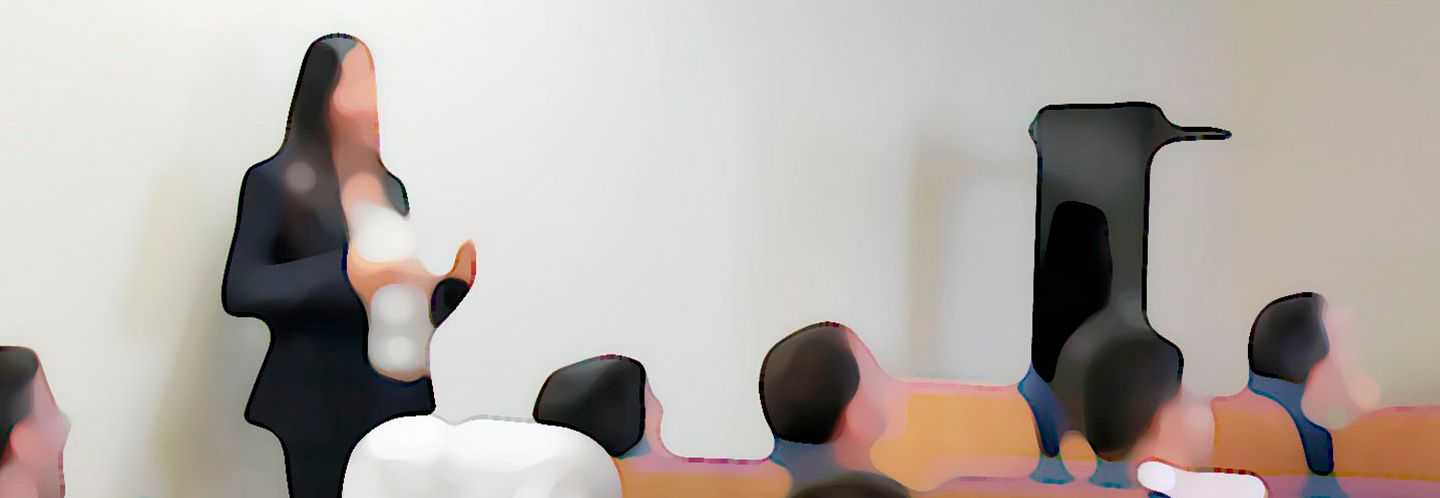
Doctoral Meeting: 'Regular Polysemy in Large Language Models'
Contextualized language models show remarkable results in various aspects of language processing. Their inner workings, however, remain obscure due to the “black box” architecture they are based on. We apply probing experiments and insights from (cognitive and psycho-) linguistics to reveal how different language phenomena are represented in the models and to what degree they resemble human language processing. We study polysemy, a form of lexical ambiguity whereby a word is associated with multiple related senses, and focus on its regularity dimension.
- Supervisors: Marcos Garcia González and Pablo Gamallo Otero
- Moderator of this DM: Eva Cernadas García
On-site event
/events/doctoral-meeting-deep-learning-techniques-for-accurate-and-efficient-biodiversity-monitoring-with-limited-data
events_en