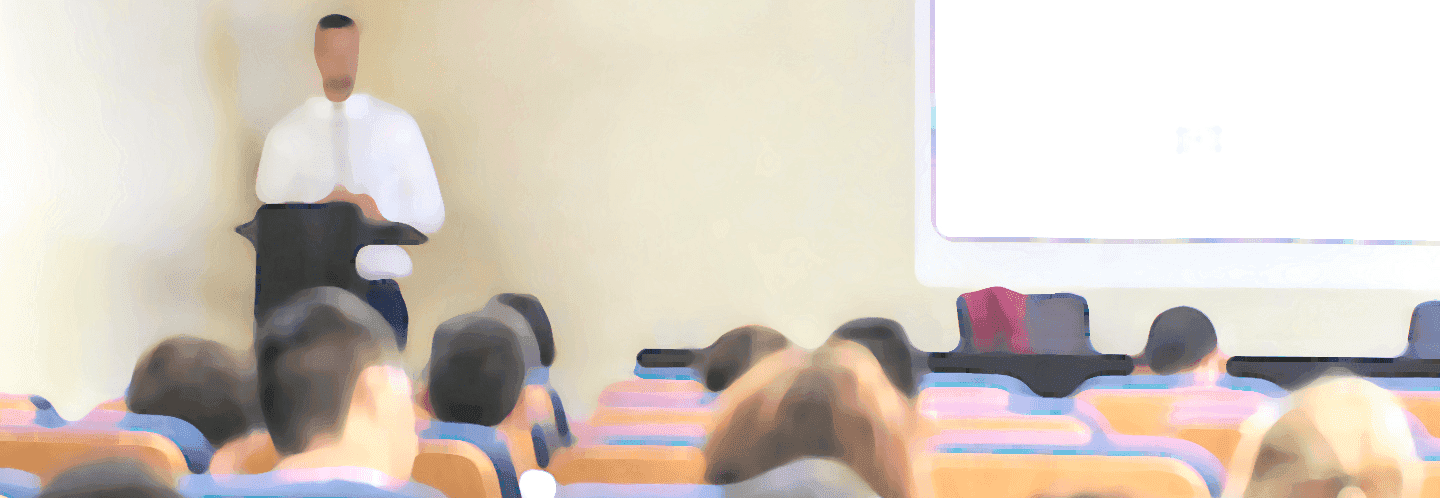
PhD Defense: 'Argumentative conversational agents for explainable artificial intelligence'
Recent years have witnessed a striking rise of artificial intelligence (AI) algorithms that are able to show outstanding performance. However, it is oftentimes achieved at the expense of explainability, which may be crucial, for example, in case of high-stakes decisions. In this thesis, we advocate the use of interpretable rule-based models that can serve as stand-alone applications or as proxies for black-box models. More specifically, we design an explanation generation framework that outputs textual factual and counterfactual explanations for predictions of interpretable rule-based classifiers following recent requirements to automated explanations. In addition, we model an argumentative communication channel between the explainer and the explainee based on insights from argumentation theory. To validate all the proposed algorithms, we carry out a series of human evaluation studies. The experimental results (1) show that the resulting interactive explanations are considered appealing by a wide range of users and (2) pave the way for adjusting the concept of counterfactual explanation to make a shift from explainable AI to trustworthy AI".
Supervisors: José María Alonso Moral & Alejandro Catalá Bolós
On-site event
/events/phd-defense-argumentative-conversational-agents-for-explainable-artificial-intelligence
events_en