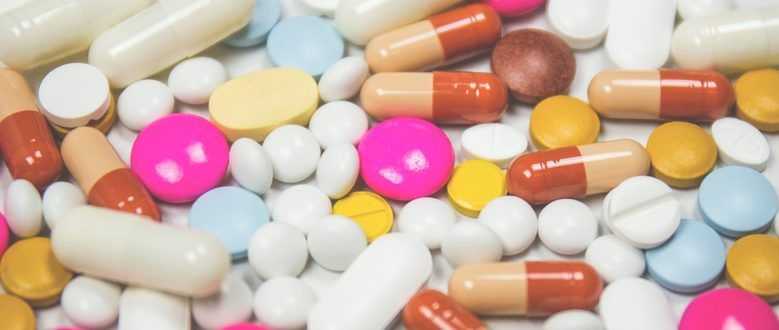
ADHERE-U: Models, techniques and methodologies based on Artificial Intelligence for improving medication adherence
The lack of medication adherence poses a key challenge for public health systems, raising a significant burden in terms of morbility, disability and mortality, and causing progressively unaffordable costs, especially affecting those people suffering from chronic conditions. This correlates with a general population ageing.
The main aim of this project is to design and develop a set of models, techniques and metodologies, based on Artificial Intelligence, in order to adaptively adjust the strategies of medication adherence to patients' behaviour, their mood, their functional limitations, and to the progress of their disease, with the ultimate purpose of improving the fulfilment of the prescribed treatment and thus makinf it more effective.
Findings and conclusions of this project will be supported by a rigurous assessment and evaluation process. Therefore, beyond the validation and evaluation of algorithms, as it is implicitly assumed in every engineering process, the present project plans to assess and evaluate the impact of the expected results on the quality of life of patients, the effectiveness of the treatment, and the costs for the public health system. At this point, we should not forget that every technological innovation entails an initial deployment cost. In this sense, a set of evaluation scenarios are proposed, obeying the paradigm of randomized controlled clinical trial. This will make it possible to assess the real value of the intervention with respect to the usual procedure along the multiple dimensions of the evaluation.
Objectives
More specifically, the objectives of the project are the following:
1) To propose, design and develop new temporal data mining procedures based on temporal constraint networks, with three specific challenges: i) representation of temporal context; ii) representation of negation; and iii) representation of calendar primitives. And with two potential applications: i) symptom pattern discovery; and ii) non-compliance pattern discovery.
2) To propose, design and develop: i) a probabilistic scheme for hypotheses evaluation in the CONSTRUE algorithm; and ii) an efficient version of this algorithm for on-line time series interpretation, by an adequate formulation of the knowledge structure based on sufficient and necessary constraints.
3) To propose, design and develop a method and system for Atrial Fibrillation detection on single lead ECG recordings.
4) To propose, design and develop a technique for emotion recognition from human computer interaction, particularly from voice interaction.
5) To propose, design and develop new explainable Artificial Intelligence algorithms so as to justify and explain in multi-modal language (combining natural language, symbols and images in an effective manner) the motivational messages as well as the suggestions delivered in accordance with patient data. We assume the higher the level of satisfaction of patients with explanations provided, the larger their confidence in the intelligent assistant, and thus the larger their medication adherence.
6) To propose, design and develop a persuasive communication strategy, based on the paradigm of Behavior Change Support Systems, in order to provide the patient and the care team with valuable information and explanations about the disease evolution, the response to treatment and, ultimately, effective information for the improvement of adherence. The communication strategy will be adapted to each user.
7) To validate and evaluate in a rigorous way those models, techniques and methodologies provided by the present project and their integration in the ADHERE-U software framework by means of a set of randomized controlled studies in different scenarios.
Project
/research/projects/modelos-tecnicas-e-metodoloxias-baseadas-en-intelixencia-artificial-para-a-mellora-da-adherencia-terapeutica
<p>The lack of medication adherence poses a key challenge for public health systems, raising a significant burden in terms of morbility, disability and mortality, and causing progressively unaffordable costs, especially affecting those people suffering from chronic conditions. This correlates with a general population ageing.<br />The main aim of this project is to design and develop a set of models, techniques and metodologies, based on Artificial Intelligence, in order to adaptively adjust the strategies of medication adherence to patients' behaviour, their mood, their functional limitations, and to the progress of their disease, with the ultimate purpose of improving the fulfilment of the prescribed treatment and thus makinf it more effective.<br />Findings and conclusions of this project will be supported by a rigurous assessment and evaluation process. Therefore, beyond the validation and evaluation of algorithms, as it is implicitly assumed in every engineering process, the present project plans to assess and evaluate the impact of the expected results on the quality of life of patients, the effectiveness of the treatment, and the costs for the public health system. At this point, we should not forget that every technological innovation entails an initial deployment cost. In this sense, a set of evaluation scenarios are proposed, obeying the paradigm of randomized controlled clinical trial. This will make it possible to assess the real value of the intervention with respect to the usual procedure along the multiple dimensions of the evaluation.</p><p>More specifically, the objectives of the project are the following:<br />1) To propose, design and develop new temporal data mining procedures based on temporal constraint networks, with three specific challenges: i) representation of temporal context; ii) representation of negation; and iii) representation of calendar primitives. And with two potential applications: i) symptom pattern discovery; and ii) non-compliance pattern discovery.<br />2) To propose, design and develop: i) a probabilistic scheme for hypotheses evaluation in the CONSTRUE algorithm; and ii) an efficient version of this algorithm for on-line time series interpretation, by an adequate formulation of the knowledge structure based on sufficient and necessary constraints.<br />3) To propose, design and develop a method and system for Atrial Fibrillation detection on single lead ECG recordings.<br />4) To propose, design and develop a technique for emotion recognition from human computer interaction, particularly from voice interaction.<br />5) To propose, design and develop new explainable Artificial Intelligence algorithms so as to justify and explain in multi-modal language (combining natural language, symbols and images in an effective manner) the motivational messages as well as the suggestions delivered in accordance with patient data. We assume the higher the level of satisfaction of patients with explanations provided, the larger their confidence in the intelligent assistant, and thus the larger their medication adherence. <br />6) To propose, design and develop a persuasive communication strategy, based on the paradigm of Behavior Change Support Systems, in order to provide the patient and the care team with valuable information and explanations about the disease evolution, the response to treatment and, ultimately, effective information for the improvement of adherence. The communication strategy will be adapted to each user.<br />7) To validate and evaluate in a rigorous way those models, techniques and methodologies provided by the present project and their integration in the ADHERE-U software framework by means of a set of randomized controlled studies in different scenarios.</p> - Paulo Félix Lamas, José María Alonso Moral - Senén Barro Ameneiro, Alejandro Catalá Bolós, Jesús María Rodríguez Presedo
projects_en