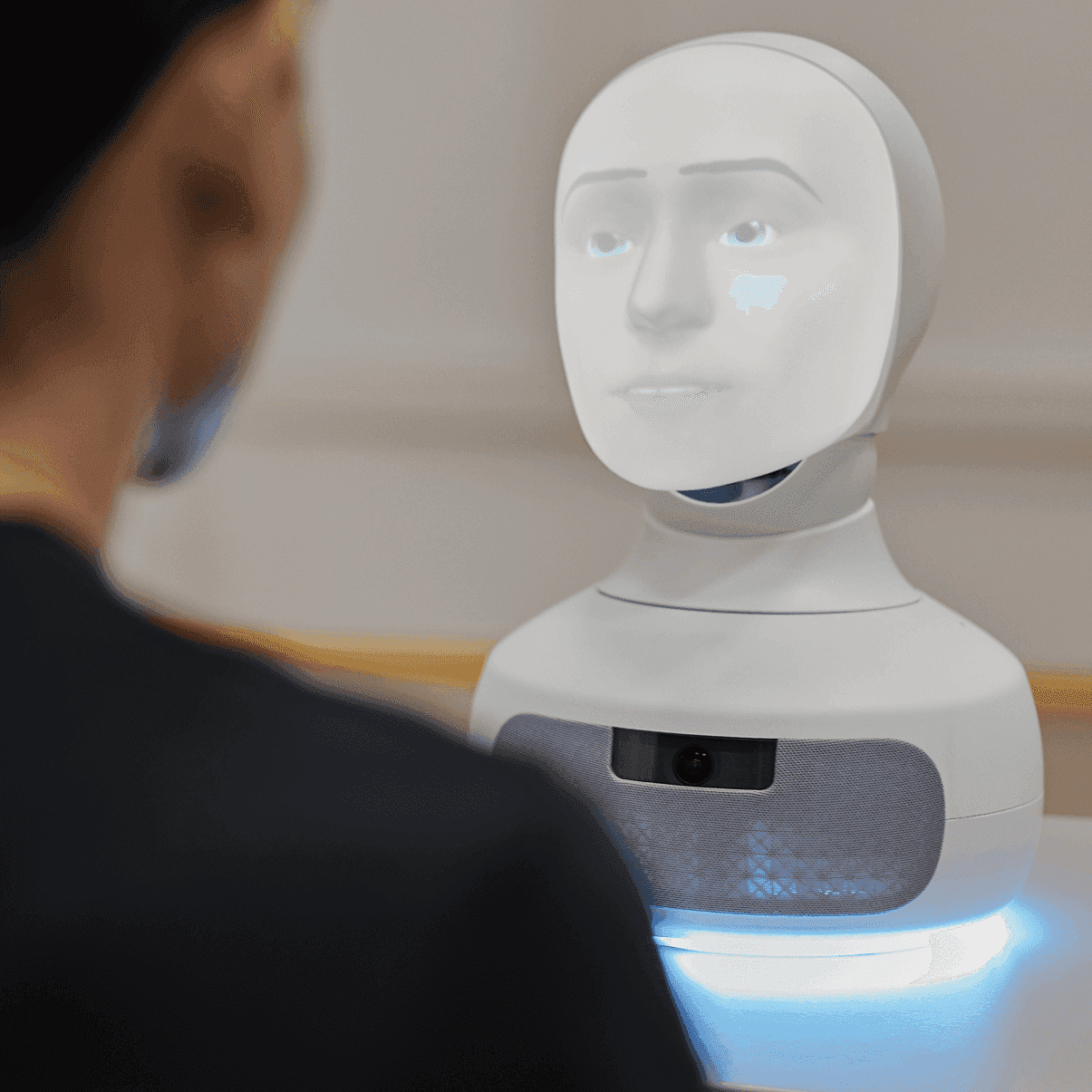
LINNA: Towards a linguistic-based inductive bias for neural language modeling
Current language models (LMs) based on artificial neural networks are able to generate high-quality text, and perform remarkably well in a variety of language processing tasks. The fact that these models are trained on simple objectives, such as predicting words in context, and that the generated text is not a simple copy of the training data, has revived the classic nature-nurture debate. On the one hand, cognitive scientists consider that current LMs implement genuine theories of language, while on the other hand, an opposing position claims that LMs are merely computational tools of no value for the scientific study of human language. In an intermediate position, several researchers from different fields argue that while these computational tools are not plausible models of human language, they can be useful for cautiously informing models of how human language works, since they allow exploring the extent to which linguistic regularities can be generalized from data without explicit linguistic knowledge.
What is clear is that current LMs based on neural networks are significantly less efficient than the biological mechanism inherent in humans, which requires substantially less data to acquire natural languages. In this regard, humans possess an inductive bias that enables the efficient acquisition of linguistic regularities and poses challenges in learning structures that do not adhere to natural language principles, unlike current LMs. Crucially, in addition to the large amount of data required to computationally model human language, training current LMs involves tuning several billion parameters, resulting in extremely complex architectures and computationally expensive models. In this context, recent research has shown that a significant part of a pre-trained language model can be removed with minimal impact on its performance. In addition, it has also been shown that pre-training a model using psycholinguistic strategies can improve its alignment with reading time prediction and generalization.
Taking the above into account, we propose a novel method to create a language-independent neural network architecture (LINNA) by pruning and distilling a linguistically diverse multilingual model. The resulting weights of this language-independent architecture are used as a proxy for a linguistic-based inductive bias to train monolingual LMs using small-scale training data and strategies motivated by child language acquisition.
Project
/research/projects/para-un-vies-indutivo-de-base-linguistica-no-modelado-neuronal-da-linguaxe
<p>Current language models (LMs) based on artificial neural networks are able to generate high-quality text, and perform remarkably well in a variety of language processing tasks. The fact that these models are trained on simple objectives, such as predicting words in context, and that the generated text is not a simple copy of the training data, has revived the classic nature-nurture debate. On the one hand, cognitive scientists consider that current LMs implement genuine theories of language, while on the other hand, an opposing position claims that LMs are merely computational tools of no value for the scientific study of human language. In an intermediate position, several researchers from different fields argue that while these computational tools are not plausible models of human language, they can be useful for cautiously informing models of how human language works, since they allow exploring the extent to which linguistic regularities can be generalized from data without explicit linguistic knowledge.</p><p><br></p><p>What is clear is that current LMs based on neural networks are significantly less efficient than the biological mechanism inherent in humans, which requires substantially less data to acquire natural languages. In this regard, humans possess an inductive bias that enables the efficient acquisition of linguistic regularities and poses challenges in learning structures that do not adhere to natural language principles, unlike current LMs. Crucially, in addition to the large amount of data required to computationally model human language, training current LMs involves tuning several billion parameters, resulting in extremely complex architectures and computationally expensive models. In this context, recent research has shown that a significant part of a pre-trained language model can be removed with minimal impact on its performance. In addition, it has also been shown that pre-training a model using psycholinguistic strategies can improve its alignment with reading time prediction and generalization.</p><p><br></p><p>Taking the above into account, we propose a novel method to create a language-independent neural network architecture (LINNA) by pruning and distilling a linguistically diverse multilingual model. The resulting weights of this language-independent architecture are used as a proxy for a linguistic-based inductive bias to train monolingual LMs using small-scale training data and strategies motivated by child language acquisition.</p> - CNS2024-154902 - Marcos Garcia González
projects_en