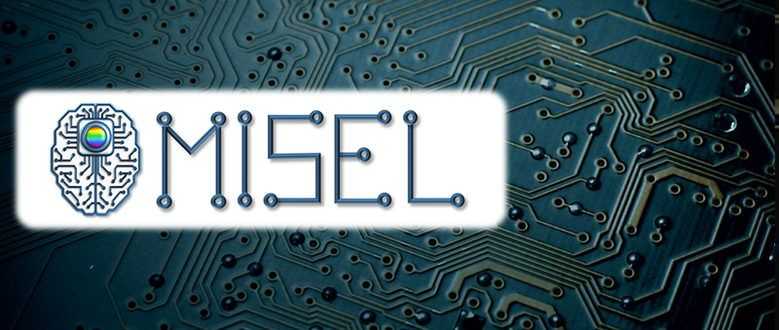
MISEL: Multispectral Intelligent Vision System with Embedded Low-power Neural Computing
MISEL aims at bringing artificial intelligence to the edge computing (decisions made on-device) through a low-power bioinspired vision system with multi-spectral sensing and in sensor spatio-temporal neuromorphic processing based on complex events. The science-to-technology breakthrough is the heterogeneous integration of a neuromorphic computing scheme featuring three different abstraction levels (cellular, cerebellar and cortex processors) with high-density memory arrays and adaptive photodetector technology for fast operation and energy efficiency. The context-aware, low power and distributed computation paradigm supported by MISEL is promising alternative to the current approach relying on massive-data transfers and large computational resources, e.g., workstations or cloud servers. This answers to the challenges and related scope presented in the Work Programme towards "more complex, brain mimicking low power systems" "exploiting a wider range of biological principles from the hardware level up" by introducing the human eye like adaptivity with cellular processor and the data fusion, learning, reasoning, and “conscious” decisions performed by the cortex.
The stand-alone system fabricated in MISEL will be tested on timely and challenging applications such as distinguishing birds from drones through their spatio-temporal flying signature, and scene anomaly detection from a mobile platform. From the technology development and industrialization point of view, MISEL includes the whole value chain: materials research for back-end of line (BEOL) processing-compatible densely-packed ferroelectric non-volatile memories (FeRAMs) and intensity adaptive photodetectors, novel neuromorphic computing algorithms and circuit implementations, and system level benchmarking. This is all in line with the challenge and scope of "outperforming conventional SoA with relevant metric" and benchmarking "challenging end-to-end scenarios of use" for industrial adaptation.
This project has received funding from the European Union’s Horizon 2020 research and innovation programme under grant agreement No 101016734
Project
/research/projects/sistema-de-vision-intelixente-multiespectral-con-computacion-neuronal-de-baixo-consumo-integrada
<p>MISEL aims at bringing artificial intelligence to the edge computing (decisions made on-device) through a low-power bioinspired vision system with multi-spectral sensing and in sensor spatio-temporal neuromorphic processing based on complex events. The science-to-technology breakthrough is the heterogeneous integration of a neuromorphic computing scheme featuring three different abstraction levels (cellular, cerebellar and cortex processors) with high-density memory arrays and adaptive photodetector technology for fast operation and energy efficiency. The context-aware, low power and distributed computation paradigm supported by MISEL is promising alternative to the current approach relying on massive-data transfers and large computational resources, e.g., workstations or cloud servers. This answers to the challenges and related scope presented in the Work Programme towards "more complex, brain mimicking low power systems" "exploiting a wider range of biological principles from the hardware level up" by introducing the human eye like adaptivity with cellular processor and the data fusion, learning, reasoning, and “conscious” decisions performed by the cortex.</p><p>The stand-alone system fabricated in MISEL will be tested on timely and challenging applications such as distinguishing birds from drones through their spatio-temporal flying signature, and scene anomaly detection from a mobile platform. From the technology development and industrialization point of view, MISEL includes the whole value chain: materials research for back-end of line (BEOL) processing-compatible densely-packed ferroelectric non-volatile memories (FeRAMs) and intensity adaptive photodetectors, novel neuromorphic computing algorithms and circuit implementations, and system level benchmarking. This is all in line with the challenge and scope of "outperforming conventional SoA with relevant metric" and benchmarking "challenging end-to-end scenarios of use" for industrial adaptation.</p><p>This project has received funding from the European Union’s Horizon 2020 research and innovation programme under grant agreement No 101016734</p> - Víctor Manuel Brea Sánchez - Diego Cabello Ferrer, Paula López Martínez, Óscar Pereira Rial, Daniel García Lesta
projects_en